Label Images for Object Detection: Unlocking the Power of Data Annotation
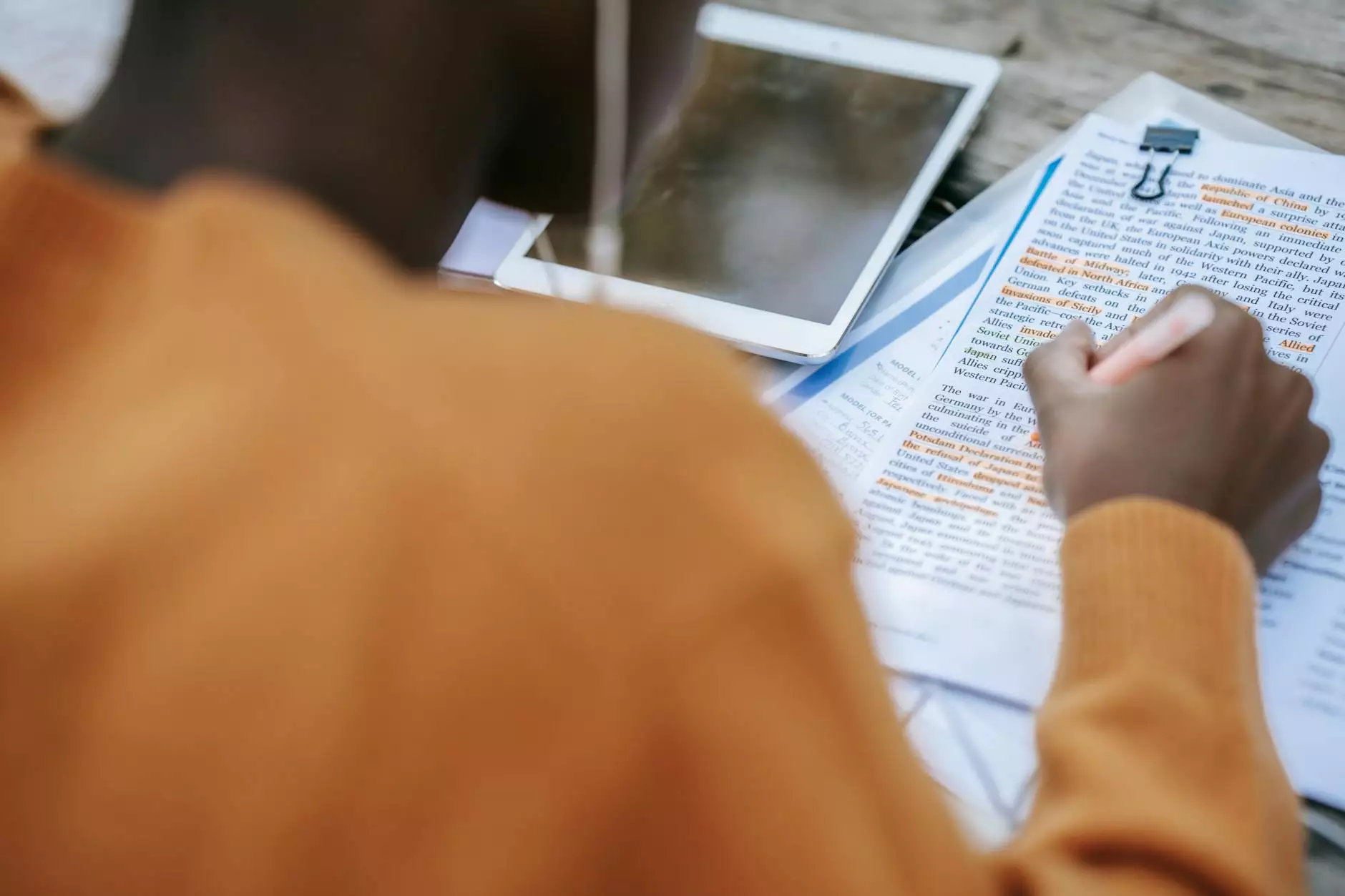
In the ever-evolving landscape of artificial intelligence (AI), the importance of quality data cannot be overstated. The foundation of any successful AI model lies in its ability to effectively detect objects in images. To achieve this, labeling images for object detection is a crucial step that cannot be overlooked. At KeyLabs.ai, we understand the nuances of data annotation and the immense potential it holds for businesses looking to leverage AI effectively. In this comprehensive guide, we will explore the intricacies of image labeling, the tools available, and how our Data Annotation Platform can transform your AI projects.
The Significance of Data Annotation in AI
Data annotation is the process of labeling or tagging data to make it usable for machine learning models. This is particularly vital in the domain of computer vision, where models must learn to recognize and interpret visual elements accurately. By labeling images for object detection, we provide the necessary annotations that enable AI systems to identify and classify visual inputs.
Understanding Object Detection
Object detection is a key task in computer vision that involves both localization and classification of objects within an image. For instance, when an AI model analyzes an image containing multiple items—such as a street scene, an indoor setting, or a natural landscape—it must not only identify what those objects are (e.g., cars, pedestrians, trees) but also pinpoint their exact locations.
To accomplish this, the process of labeling images becomes essential. Each object needs to be annotated with a specific label and, often, parameters defining its bounding box. Hence, the necessity for detailed and accurate data annotation processes is paramount for high-performing AI systems.
Why Choose KeyLabs.ai for Data Annotation?
At KeyLabs.ai, we offer a cutting-edge Data Annotation Tool designed to simplify and enhance the image labeling process. But what truly sets us apart? Let’s delve into the features and advantages of our platform:
- User-Friendly Interface: Our platform is designed with users in mind. Whether you're a seasoned data scientist or a novice, you can navigate our tools easily.
- Scalability: We cater to projects of all sizes. From small datasets to enterprise-scale requirements, our solutions can scale accordingly.
- Quality Assurance: Accuracy is critical in data annotation. Our platform incorporates verification steps to ensure high-quality annotations.
- Customizable Solutions: Every project is unique; we provide customizable annotation options to meet the specific needs of your applications.
- Rapid Turnaround Time: Speed is essential in the fast-paced tech world. Our efficient processes ensure timely delivery of annotated datasets.
The Process of Labeling Images for Object Detection
Labeling images for object detection involves several key steps. Here’s a detailed breakdown of the process:
Step 1: Image Collection
The first stage in the image labeling workflow is gathering a diverse dataset. The quality and variety of images significantly impact the accuracy of the trained model. This can include images from various angles, lighting conditions, and backgrounds.
Step 2: Preprocessing Images
Once images are collected, they may require preprocessing. This can involve resizing, cropping, and enhancing images to ensure they are suitable for accurate annotations. Preprocessing helps maintain consistency across the dataset, which is crucial for effective training of AI models.
Step 3: Annotation
During the annotation phase, each image is reviewed, and objects of interest are labeled accordingly. This involves creating bounding boxes around objects and assigning appropriate labels. Here's where a powerful data annotation tool like the one from KeyLabs.ai shines, enabling quick and precise labeling.
Step 4: Quality Check
Quality control is an essential step in the labeling process. After annotations are completed, a secondary review should ensure that all labels are accurate and consistent. Employing multiple annotators and cross-checking can further enhance accuracy.
Step 5: Integration and Testing
After the images are labeled and quality-checked, it’s time to integrate the annotated dataset into your machine learning model. It's important to conduct extensive testing to assess the model's performance on the new data. This feedback loop is crucial for ongoing model improvement.
Challenges in Labeling Images for Object Detection
While the job of labeling images seems straightforward, several challenges can arise:
- Complexity of Scenes: Images with multiple overlapping objects can complicate the annotation process. AI algorithms may struggle to differentiate between objects if annotations are not precise.
- Varying Object Characteristics: Different objects may have similar appearances or can be obscured in certain images, making accurate labeling a challenge.
- Scalability Issues: Manually labeling images can be time-consuming. High-volume projects can quickly become unmanageable without the right tools.
Best Practices for Effective Image Labeling
To overcome challenges and ensure successful labeling, consider the following best practices:
- Define Clear Guidelines: Establishing clear annotation guidelines helps annotators understand how to label objects consistently. This includes defining classes, bounding box parameters, and acceptable visibility of objects.
- Use a Collaborative Annotation Tool: Enable your team to work together through collaborative tools that allow real-time feedback and communication.
- Leverage Automation: Incorporate AI-powered tools to assist in the initial labeling process to speed up and enhance consistency.
- Regular Training Sessions: Conduct training sessions for your annotators to keep them updated on best practices and new strategies in object detection.
Future of Data Annotation in AI
The future of data annotation in AI is promising, with advances in technology continually pushing the boundaries of what is possible. Techniques such as semi-supervised and unsupervised learning are evolving, thereby reducing the reliance on extensive labeled datasets. Here’s what to keep an eye on:
- Automated Annotation Tools: The rise of machine learning-based annotation tools will increasingly assist in labeling processes, thereby increasing efficiency.
- Interactive Tools: Future annotation platforms may incorporate more interactive features, allowing for better collaboration and feedback loops.
- Integration with AI Workflows: Data annotation will be more seamlessly integrated into AI workflows, providing real-time labeling capabilities as new data becomes available.
Conclusion
In conclusion, the ability to label images for object detection is a cornerstone of successful AI applications. The accuracy and quality of labeled data are critical in determining the performance of AI models. At KeyLabs.ai, we provide powerful data annotation tools and platforms that simplify this process and empower businesses to harness the full potential of their data.
By adopting the right strategies and leveraging advanced technologies, companies can not only improve their object detection capabilities but also gain a competitive edge in their respective industries. Whether you’re starting your journey in AI or enhancing existing projects, a robust data annotation solution is essential for success.