Labeling Training Data: The Backbone of Successful AI Models
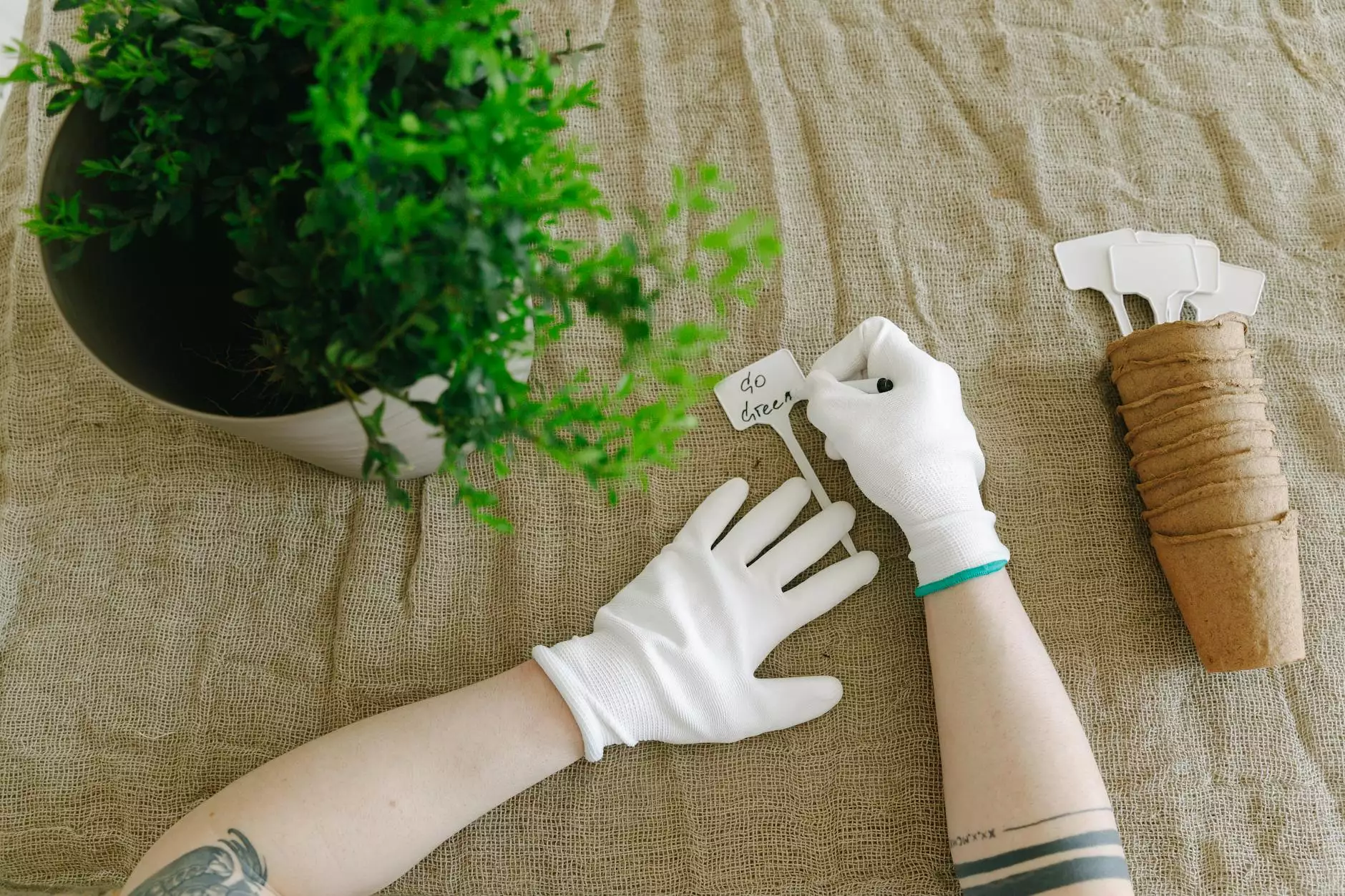
Labeling training data is a pivotal aspect of the machine learning and artificial intelligence development process. As businesses increasingly depend on AI and machine learning technologies, the significance of high-quality labeled data has never been more pronounced. In this article, we will explore the intricacies of labeling training data, its implications on business, and how using advanced tools and platforms like those from Keylabs.ai can elevate your projects.
Understanding Labeling Training Data
At its core, labeling training data involves the process of annotating or tagging data to provide context, which allows machine learning models to learn from the information provided. Essentially, without proper labeling, your AI models lack the foundational knowledge necessary to understand patterns and make predictions.
Why is Labeling Training Data Important?
The importance of labeling training data cannot be overstated. Here are some key reasons:
- Enhances Model Accuracy: Accurate labels directly impact the performance of AI models. Well-labeled data helps algorithms to discern between different classes and make informed decisions.
- Enables Supervised Learning: Labeling is crucial for supervised learning, where the algorithm learns from a labeled dataset to make future predictions.
- Facilitates Debugging and Refinement: Labeled datasets allow data scientists to evaluate model performance and make necessary adjustments to improve accuracy.
- Provides Accountability and Traceability: Proper labeling ensures that data can be traced back to its origin, vital for adhering to compliance and ethical standards.
Types of Data that Require Labeling
Various types of data require labeling for effective analysis and training. Here are a few common data types:
Image Data
In computer vision applications, labeling training data involves tagging images with specific categories or identifying objects within the images. For example, labeling images of animals may involve categorizing them as cats, dogs, or birds.
Text Data
For natural language processing (NLP), labeling training data can include sentiment analysis, where text is classified as positive, negative, or neutral, among other categorizations.
Audio Data
In speech recognition systems, label training data can involve identifying spoken words and phrases within audio recordings, essential for teaching models to understand human speech effectively.
Time Series Data
Financial forecasting and other applications may involve labeling data points with specific time stamps and identifying patterns over time, essential for accurate predictions.
Challenges in Labeling Training Data
Despite its importance, labeling training data comes with its challenges:
- Resource-Intensive: The process can be time-consuming and often requires significant human resources to ensure accuracy.
- Subjectivity: Different annotators may label the same data differently, leading to inconsistencies in datasets.
- Scalability Issues: As the volume of data increases, scaling the labeling process can become overwhelming.
- Quality Control: Maintaining high quality in labeling requires ongoing training and supervision of annotators, especially as datasets evolve.
How Keylabs.ai Revolutionizes Labeling Training Data
To tackle the challenges of labeling training data, using a robust data annotation platform such as Keylabs.ai can significantly enhance your data management processes. Here’s how:
Superior Annotation Tools
Keylabs.ai provides advanced annotation tools that simplify the labeling process, allowing you to annotate images, text, and audio with precision. These tools are designed to accommodate various data types and support multiple labeling tasks, thus enhancing the overall productivity of your team.
AI-Assisted Annotation
Utilizing machine learning algorithms, Keylabs.ai offers AI-assisted annotation features that can automatically label portions of your data, allowing human annotators to focus on refining and correcting labels rather than starting from scratch. This hybrid approach drastically reduces time and costs associated with data labeling.
Quality Assurance Processes
To ensure the accuracy of your labeled data, Keylabs.ai implements rigorous quality assurance (QA) processes. Automated audits and human review cycles help identify and rectify labeling errors, ensuring that your models are trained on the highest quality datasets.
Scalability and Flexibility
Keylabs.ai's platform is designed for scalability. Whether you're working with a small dataset or a massive project, the platform can easily adapt to your needs. This flexibility allows businesses of all sizes to leverage effective data labeling without compromising on quality.
Best Practices for Labeling Training Data
To maximize the effectiveness of your labeled datasets, consider implementing the following best practices:
- Define Clear Guidelines: Establish clear labeling guidelines to reduce inconsistencies among annotators. Detailed instructions help ensure everyone understands the labeling criteria.
- Invest in Training: Providing comprehensive training for annotators is vital. This prepares them for the nuances of the labeling process and reduces errors.
- Regularly Review and Update Data: As new data emerges, there may be a need to revisit previously labeled data to maintain accuracy and relevance.
- Utilize a Diverse Annotator Pool: Having a diverse group of annotators can enhance the understanding of data context and increase the richness of annotations.
Case Studies: The Impact of Effective Labeling
The impact of effective labeling training data is evident in various industries. Here are some noteworthy examples:
Healthcare
In medical imaging, accurate labeling of X-rays and MRIs has significantly improved the accuracy of diagnostic algorithms. For instance, AI models trained with well-labeled imaging data assist radiologists in diagnosing diseases more effectively, reducing human error.
Retail
Using labeled data from customer interactions, retailers implement machine learning models to predict consumer trends and personalize marketing efforts. Well-labeled datasets enable retailers to understand what products appeal to specific demographics, boosting sales.
Autonomous Vehicles
Self-driving technology relies heavily on accurately labeled sensory data from cameras and lidar. This labeled training data teaches AI systems to recognize road signs, pedestrians, and other vehicles, paramount for safe autonomous navigation.
The Future of Labeling Training Data
As we look toward the future, the landscape of labeling training data is expected to evolve significantly. Here are some potential trends:
- More Automation: The advancement of AI will lead to more automated labeling solutions, making the process faster and increasingly cost-effective.
- Greater Collaboration: Organizations may develop partnerships to share labeled datasets, fostering innovation and reducing individual burdens in data collection.
- Focus on Ethical AI: As the need for ethical AI grows, the emphasis on transparent labeling processes and accountable AI systems will become paramount.
Conclusion
In conclusion, labeling training data is integral to successfully developing AI models that deliver accurate predictions and insights. As businesses across various sectors leverage AI technology, the demand for effective data annotation tools and platforms, like those offered by Keylabs.ai, will continue to grow. By investing in high-quality labeled data and employing best practices, organizations can unlock the true potential of their AI initiatives, leading to innovation and competitive advantage in their markets.
Let Keylabs.ai be your partner in navigating the complexities of data annotation. Together, we can ensure that your AI projects are built on a solid foundation of high-quality labeled training data.